03 Dec 2021
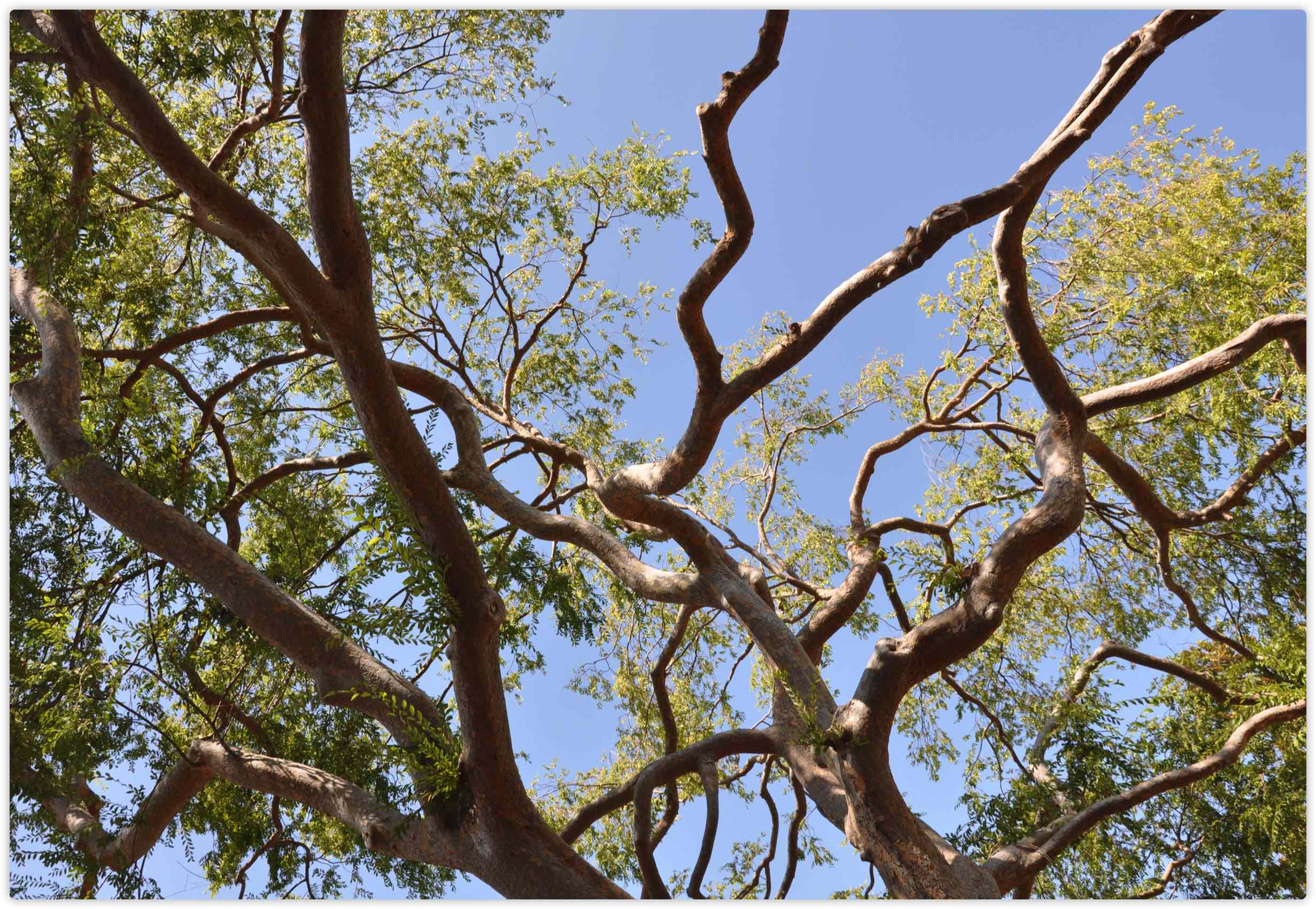
A data model describes the data with objects and relationships.
Document model was used in IBM’s Information Management System (IMS), the popular database for business data processing in the 1970s. It did quite well when the relationship among objects is one-to-many. When more complex relationships like many-to-many are involved, normalization is necessary to eliminate the duplicates and JOIN is required for data combination. However the document model can support neither normalization nor JOIN, in the efficient way. Two models were introduced to address the challenge by many-to-many relationships: the network model and the relational model.
The network model is a natural generalization of hierarchical or tree structure of the document model. In the network model, an object can link to more than one child and be linked from multiple parents; therefore many-to-one or many-to-many relationships are allowed. To access a record or object, an access path must be provided to follow a path from a root record along these chains of links. It is possible for many paths to reach the same object if many-to-many relationships exist in the network. The developer should keep all these in mind while retrieving any records. Thus the complexity of querying and updating the data can be a huge concern. The idea died down after 1980s.
On the other hand, the relational model flattens the data into a set of tables (or relations), each of which has a collection of rows (or tuples). The key and foreign key are introduced as an attribute or field into tables to build the relationships. JOIN is supported to combine relations with the foreign keys in the query execution. Conceptually it still needs the access path to query any records in the database, but implicitly. The query optimizer can automatically choose the right and shortest path to execute the query. User doesn’t have to worry about how the query optimizer works and can focus on the business logic mostly. Surprisingly the relational model thrives decades and until now it dominates the database world.
There is a chapter in the book, Designing Data-Intensive Applications with an overview of the data models and query languages. Here I presented the chapter, giving more details about the above.
20 Nov 2021
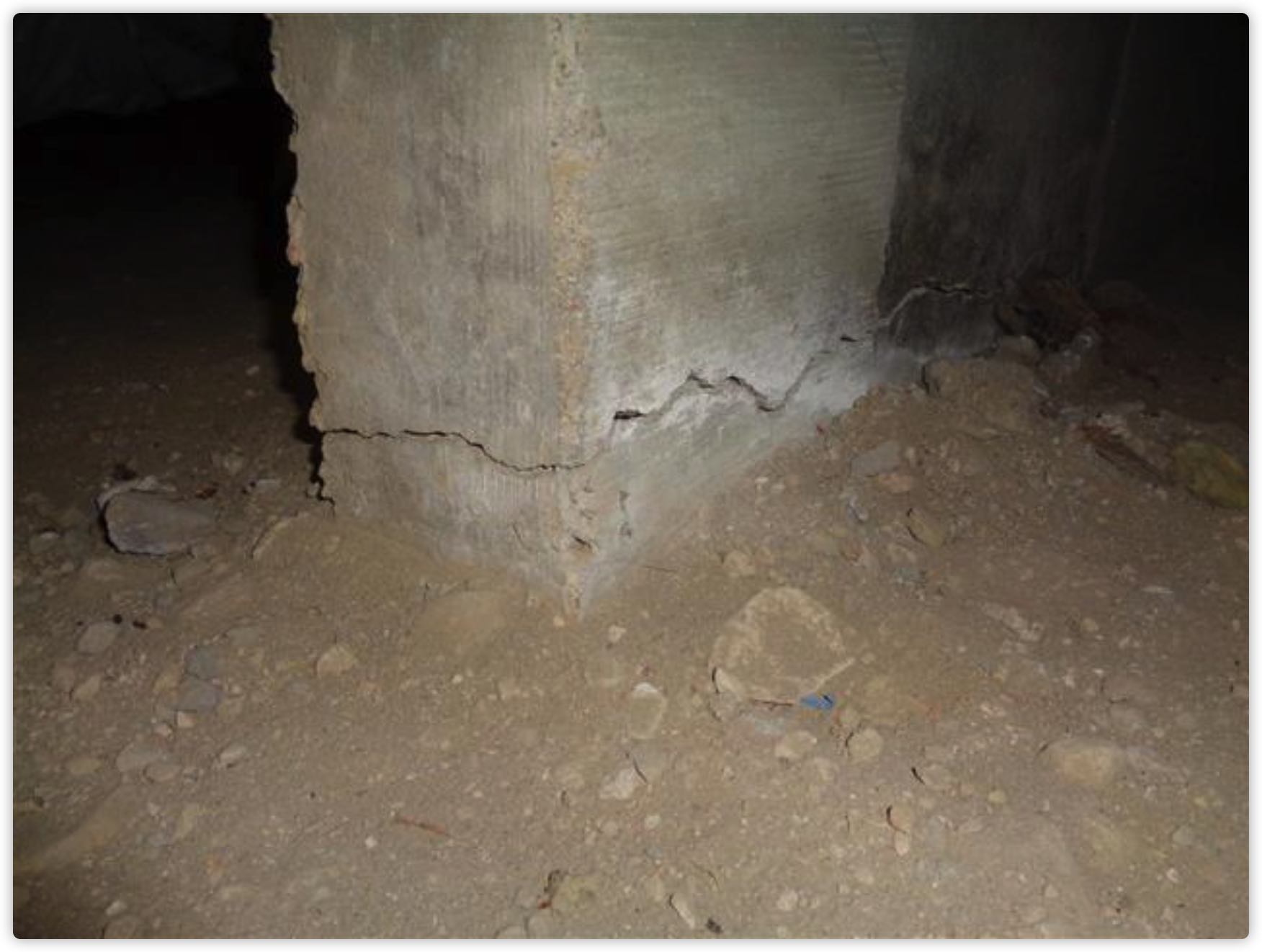
A successful data system should be able to meet various requirements while solving the data problems, including functional requirements and nonfunctional requirements.
The functional requirements are often application specific, describing what to be done with the data and how the results can be achieved.
In the nonfunctional requirements, there are many factors affecting the design and implementation, wherein three aspects are so important that all should consider throughout the development cycle: reliability, scalability and maintainability.
Any data system is a software developed by humans, deployed and run in the environment composed of hardwares. It is important to make the system work correctly even when faults occurs. The problem can be caused by hardware faults (e.g., network interruption, disk failure, power outage), software issues (e.g., bugs) and human errors (e.g., misconfiguration, mistakes in operation). Reliability introduces the concept and a guidance to exploit fault-tolerance techniques.
In the real world, the data system grows as it has an input with larger data or traffic volume, often more complex. We need a precise measurement of load and performance, based on which the strategies can be taken to keep performance constant, therefore achieve good scalability.
Additionally a data system often has a long life cycle, thus its maintainability plays a critical role in the course of evolution. As it suggests, “good operations can often work around the limitations of bad software, but good software cannot run reliably with bad operations”. Both engineering and operations teams ought to work together, sometimes grow with the system.
The book Designing Data-Intensive Applications gives a good discussion and helps with a guidance in the design of data system with reliability, scalability and maintainability considered. Here I presented the first chapter, as the start of a long journey.
24 Jul 2021
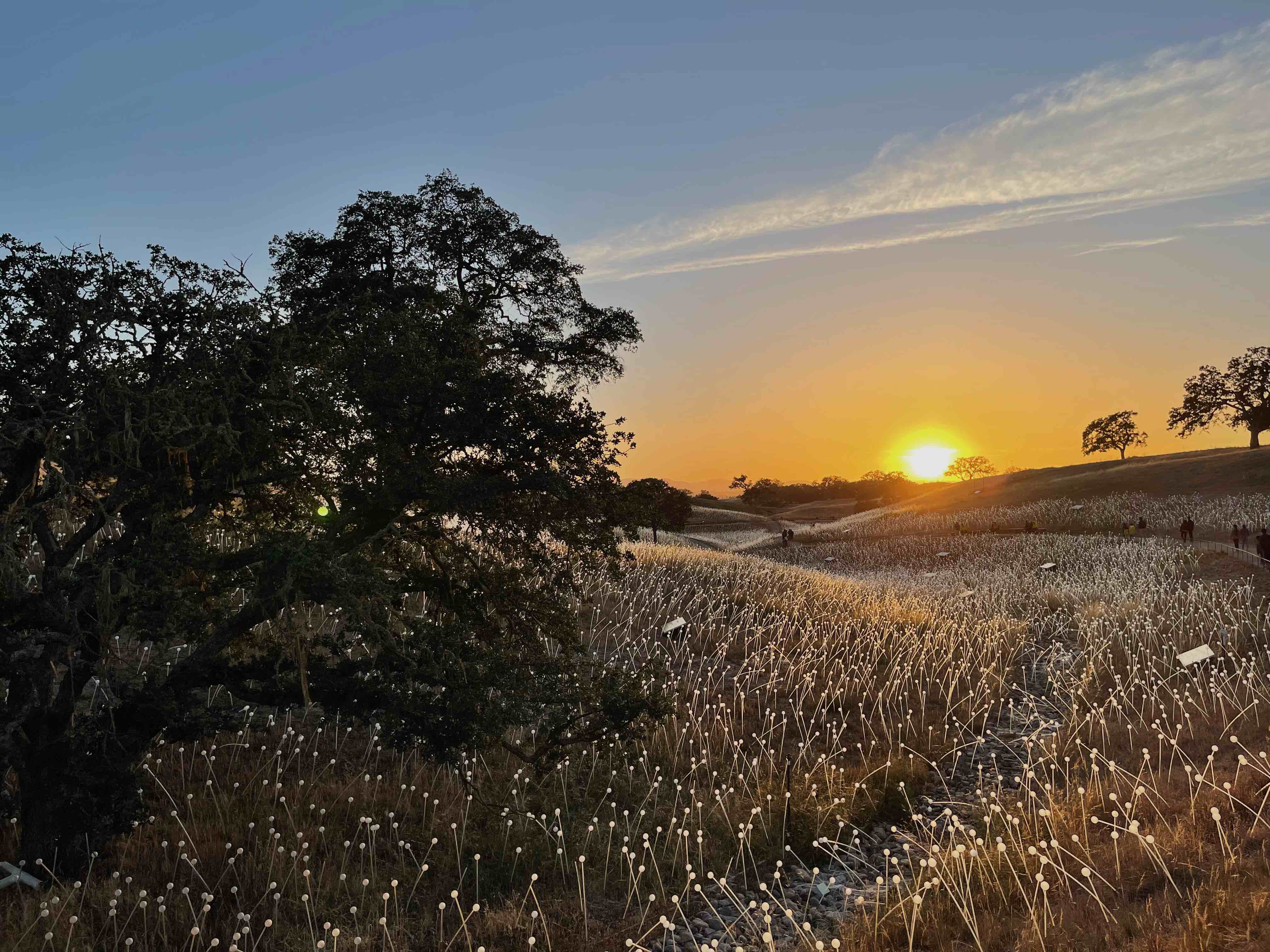
In the past 20 years, the human life expectancy has been improved significantly, and the retirement age has been rising. In other words, the retirement is starting later but lasting longer. People used to think that careers would be over when they are around 40s. However, it may not be even at the halfway point. Actually people tend to underestimate the length of a career. Therefore it is necessary to plan for a long career journey, especially if a successful career is concerned.
Generally careers can be divided into three stages:
- Start strong in the first 15 years of the career;
- Reach high in the middle;
- Go far near or even beyond retirement.
The book The Long View tries to introduce us to a set of career mindset, framework, and tools, to help us learn how to collect the ‘fuel’ to achieve our career goals at the different stages. As a result of reading and learning, I made a presentation based on the book, hopefully it could highlight the main points.
22 Jun 2021
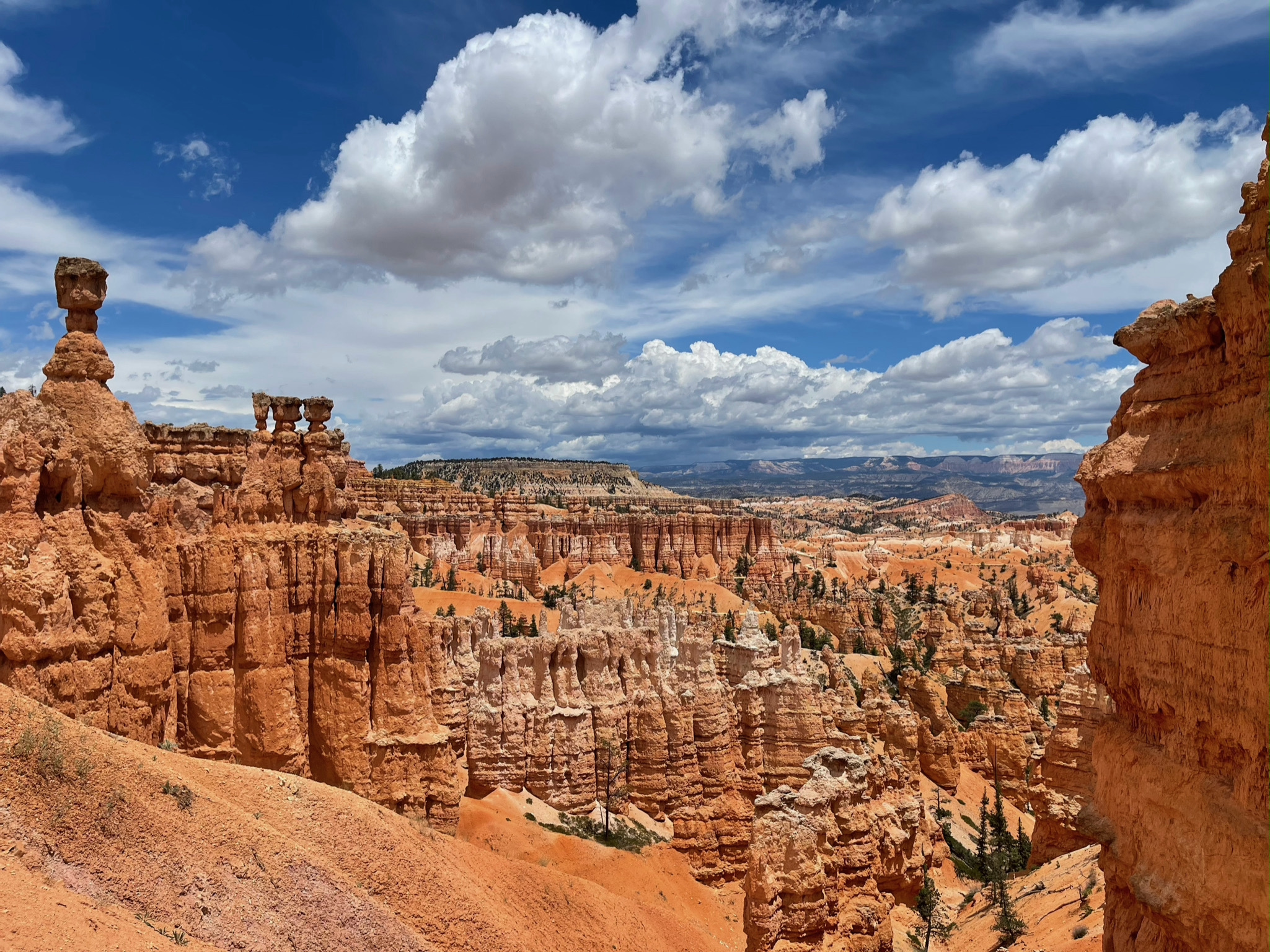
Software development has many similarities with building construction. There are a few of rules that seem simple, like the physics of gravity in the real physical world or the single responsibility principle (SRP) in the programming. However, not all developers can necessarily use them well, especially in the complex scenario. An architect should have a good understanding of those principles first, and grow a pair of sharp eyes to see through the complexity, such that she can apply those rules to achieve a clean architecture.
Uncle Bob in his book, Clean Architecture: A Craftman’s Guide to Software Structure and Design gives a detailed description on those principles. More importantly he tries to explain how clean architecture can be achieved with the help of them. As a result of reading and learning, I made a presentation based on the book, hopefully it could highlight the main points.
12 Oct 2020
Teamwork is everywhere, especially important in the human society. For example in the software project, it cannot be emphasized too much as long as more than one person get involved. There can be many aspects affecting the performance of teamwork. The keys are about communication and collaboration.
In my not-so-long career as software engineer, I found one of biggest challenges that prevent developers from delivering a successful software product is due to the communication gap between them and their business partners. Many failures could be avoided if both parties are able to sync-up earlier. However, timing is not the only factor. The communication may lead nowhere if the common language is absent. The business people often use a human language, like English to describe what they need, or the specifications; whereas, developers prefer more formal languages, typically thinking of translating the business specifications into code (e.g., acceptance tests). This difference clearly causes a challenge.
Agile tries to address the challenge faced by a small group of software developers with a feedback-driven approach. Therefore a software project is composed of many small cycles, each of which aims to provide a working or deliverable product that their business partners can review and both sides would discuss and decide what to do next. Instead of particular rules or steps, Agile emphasizes a set of principles and values, and encourages to cultivate a culture out of those. The book by Robert C. Martin, Clean Agile: Back to Basics gives a very clear explanation on these values and principles. Furthermore, it provides quite a few guides for applying Agile in practice. As a result of reading and learning, I made a presentation based on the book, hopefully it could highlight the main points.